Diagnostic System Based on Deep Learning to Detect Diabetic Retinopathy
Doi: 10.36351/pjo.v40i3.1771
DOI:
https://doi.org/10.36351/pjo.v40i3.1771Abstract
Purpose: To develop a machine learning based diabetic retinopathy screening system to help ophthalmologists for initial level screening.
Study Design: Diagnostic accuracy study.
Place and Duration of Study: Haldwani in a private hospital from January, 2023 to June, 2023.
Methods: A total of 229 fundus images (people suffering from diabetic retinopathy)were used which had micro aneurysms, soft exudates, hard exudates and hemorrhages. We classified these images and pre-processed them by scaling, orienting, and color adjustments. With the help of various pre-processing techniques, we decreased the size of our dataset so that it can be handled efficiently by our model with optimal resources.Visual Geometry Group (VGG) is a type of pre-trained deep convolutional neural network (CNN). The term “deep” refers to the number of layers; the VGG-16 uses 16 and VGG-19 uses 19 convolutional layers respectively. The model was tested on fresh retinal dataset.
Results: Our research has demonstrated promising results, achieving a high accuracy rate of 90% on a human dataset by utilizing VGG16 for feature extraction and a Logistic Regression classifier for classification.
Conclusion: Ophthalmologists can utilize this machine learning based screening system for diabetic retinopathy screening.
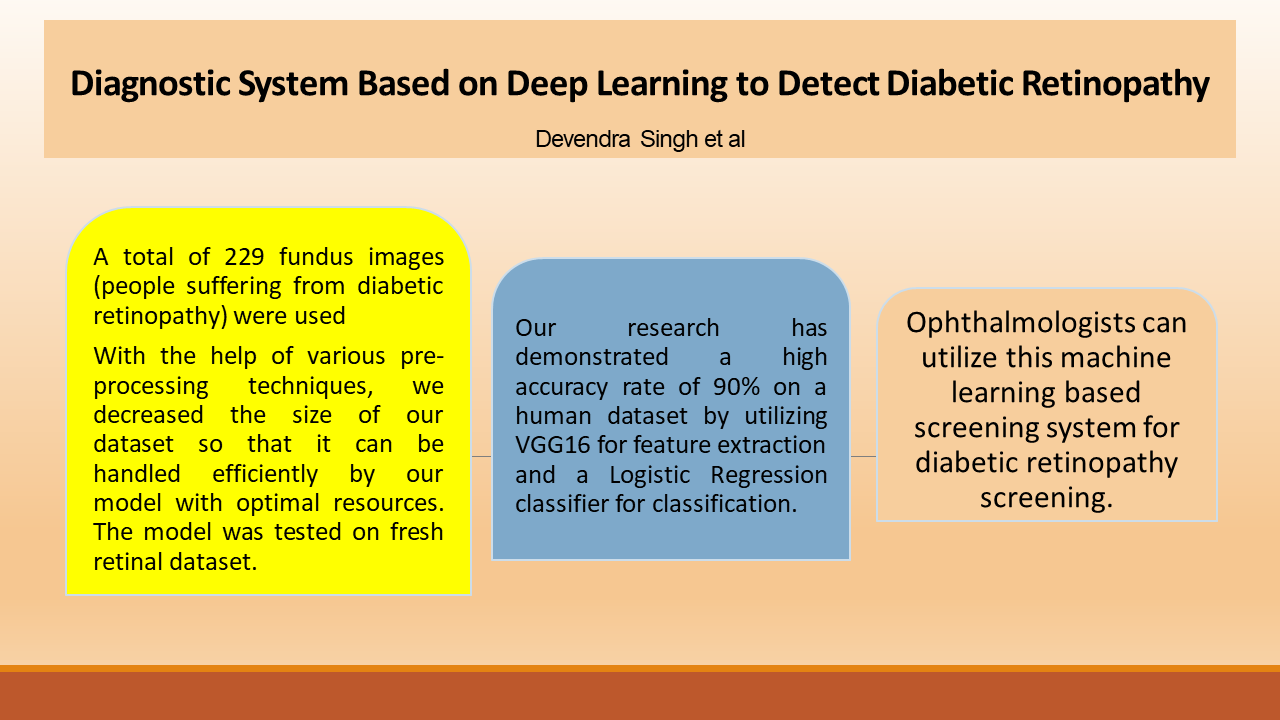
Downloads
Published
How to Cite
Issue
Section
License
Copyright (c) 2024 Devendra Singh

This work is licensed under a Creative Commons Attribution-NonCommercial 4.0 International License.